Recently, the second CCF Origin Pilot Cup Quantum Computing Challenge, which lasted for over three months, came to a close. Led by Professor Gao Fang from School of Electrical Engineering and the Key Laboratory of Intelligent Control and Operation & Maintenance of Electric Power Equipment in Guangxi, the GXU-LIPE-Q1 team achieved the Special Prize in the Professional Group’s Quantum Biotechnology and Chemical Engineering section. In the mean while, the GXU-LIPE-Q2 team secured the First Prize in the Professional Group’s Quantum Finance, while the Guangxi University team received the Second Prize in the University Group. These distinctions placed GXU teams at the forefront among educational institutions and businesses nationwide. Professor Gao Fang was also honored with the Outstanding Supervisor Award for his guidance throughout the competition.
This conference will serve as a platform to showcase the latest achievements in quantum computing in digital China, share development experiences, and provide an excellent forum for interdisciplinary collaboration and innovative integration across various technological domains in the field of quantum computing. It will offer diverse perspectives on the evolving landscape of quantum computing in China. As part of the conference proceedings, an awards ceremony for the programming challenge will be conducted, during which team representative, graduate student Wu Guojian, has been invited to deliver a specialized presentation.
The GXU-LIPE-Q1 team addressed the challenge of calculating the ground-state energy of benzene ring structural units by designing a variational quantum eigenstate-solving algorithm based on bridge circuits and gradient filters. This algorithm, proposed based on the bridging effect of quantum gates, known as the LIPE-UCC ansatz, effectively reduces the quantum resource overhead by minimizing the number of quantum gates and circuit depth compared to the original UCCSD ansatz. Additionally, it employs a parameter gradient filtering strategy to reduce the number of variational parameters requiring optimization. This algorithm extensively explores the potential of LIPE-VQE for determining the ground-state energy of chemical molecules. Consequently, it achieves efficient and precise computation of the simplified ground-state energy of benzene ring molecules within a shorter timeframe, thereby demonstrating the effectiveness and excellence of LIPE-VQE.
The GXU-LIPE-Q2 team has tackled the issue of risk assessment in stock funds by introducing a Quantum Long Short-Term Memory Network (QLSTM) and developing a model for stock fund risk evaluation. This model's algorithm is rooted in the classical Long Short-Term Memory Network (LSTM), with the neural network component being substituted by a variational quantum circuit, thus establishing a versatile classical-quantum neural network framework. Using a training dataset spanning six months of historical data, QLSTM has effectively and accurately forecasted the future month’s risk levels for a variety of stock funds, surpassing LSTM in terms of precision. The successful implementation of QLSTM in stock fund risk prediction underscores its significant potential for application in other forecasting problems, while also affirming the effectiveness of this classical-quantum neural network framework.
The undergraduate team from GXU has developed a Markov-based quantum neural evolution algorithm designed to address the challenge of arbitrary quantum state preparation. This algorithm employs a quantum neural network to simulate a probability distribution and utilizes classical evolutionary algorithms to optimize this distribution, ultimately yielding the optimal solution. It represents a heuristic algorithm that combines classical optimization techniques with quantum computing principles.
The use of this algorithm in quantum circuit searches takes into full consideration the connectivity constraints of two-qubit gates. It achieves a high level of precision in arbitrary initial state preparation with a reduced usage of two-qubit gates. This approach provides a theoretical basis for the development of noisy intermediate-scale quantum devices.
The second CCF Origin Pilot Cup Quantum Computing Challenge, hosted by the China Computer Federation (CCF) and organized by the CCF Quantum Computation Professional Group in collaboration with China’s pioneering quantum computing company, Origin Quantum, featured two categories: University and Professional. Within the Professional category, there were four tracks: General, Quantum Machine Learning, Quantum Finance, and Quantum Biotechnology and Chemical Engineering. This competition attracted nearly a thousand teams from across the globe, with the Professional category alone boasting 415 teams representing nearly 150 academic institutions and 50 companies, totaling over 700 participants. It stands as the largest-scale quantum computing programming challenge in China, aiming to elevate the overall proficiency of quantum computing programming in China, identify, gather, and nurture outstanding potential talents in quantum computing programming, actively promote the development of China’s quantum computation industry, and enhance the influence of the quantum computing field in national scientific activities, societal services, and international academia.
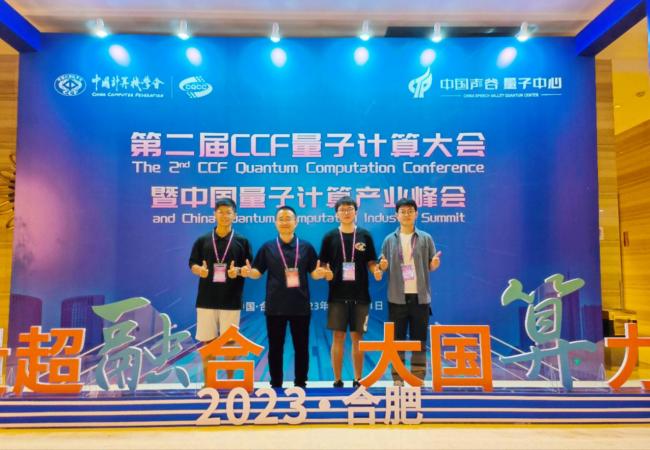